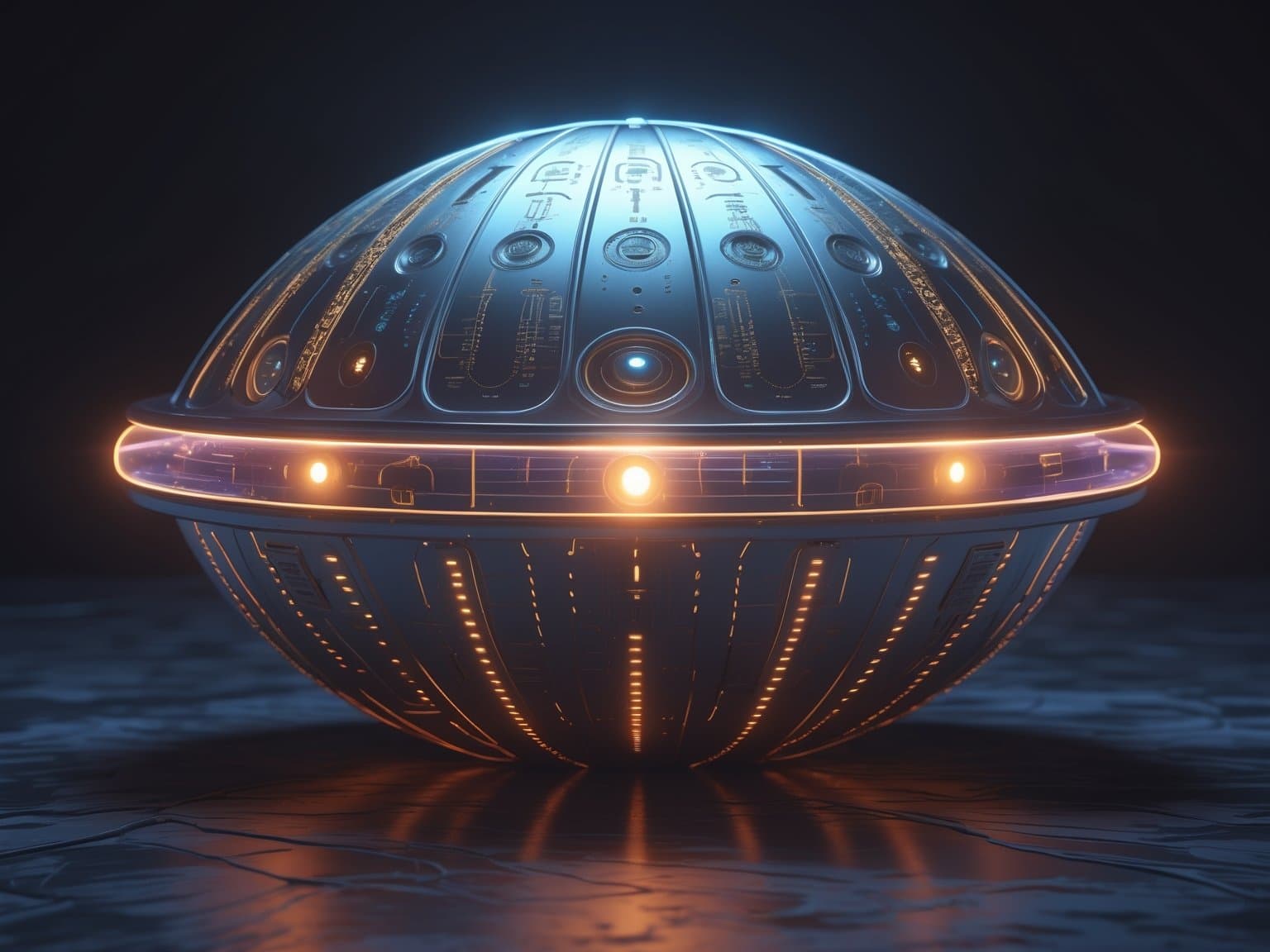
DeepSeek R1 vs OpenAI O1: AI Models Face Off
The AI landscape is witnessing an intriguing battle between DeepSeek R1 and OpenAI’s O1, two groundbreaking language models that showcase different approaches to artificial intelligence. While both push the boundaries of AI capability, they differ significantly in their architecture, specializations, and real-world applications.
Core Architecture and Training Approach
Based on the research and provided guidelines, here’s the chapter focusing on Core Architecture and Training Approach:
The architectural DNA of DeepSeek R1 and OpenAI’s O1 reveals fundamentally different approaches to artificial intelligence development, highlighting the evolving landscape of large language models. While both models represent significant advances in AI technology, their core differences extend beyond mere technical specifications to encompass broader philosophical approaches to AI development.
At the heart of DeepSeek R1’s architecture lies an impressive feat of efficiency optimization. Training the model required just $6 million in resources, a fraction of traditional development costs for models of similar scale. This cost-effective approach wasn’t merely about budget constraints; it represents a deliberate architectural choice focusing on resource-efficient training methodologies and streamlined computational pathways. R1’s architecture emphasizes open-source accessibility while maintaining competitive performance, demonstrating that cutting-edge AI development doesn’t necessarily require massive financial investment.
In contrast, OpenAI’s O1 introduces a groundbreaking ‘thinking before responding’ architecture, fundamentally changing how the model processes and generates responses. This architectural innovation implements a multi-stage cognitive pipeline where the model first constructs an internal representation of the problem space before formulating its response. The approach mirrors human cognitive processes more closely than traditional transformer architectures, representing a significant departure from conventional language model design.
The computational infrastructure between these models reveals another layer of differentiation. R1’s architecture prioritizes efficient resource utilization through:
- Optimized training data preprocessing pipelines
- Innovative parameter sharing mechanisms
- Streamlined attention mechanisms that reduce computational overhead
Meanwhile, O1’s architecture emphasizes:
- Advanced reinforcement learning integration for response refinement
- Sophisticated optimization algorithms that enhance response accuracy
- Dynamic resource allocation based on task complexity
The scaling approaches adopted by both models present another significant architectural contrast. DeepSeek R1 implements a horizontal scaling strategy that maximizes performance across distributed computing environments, making it particularly suited for deployment across varied infrastructure configurations. This approach aligns with its open-source nature, enabling broader adoption across different computational environments. O1, conversely, employs a vertical scaling methodology that leverages specialized hardware optimizations and dedicated infrastructure to achieve its performance targets.
Perhaps most intriguingly, the models diverge in their approach to knowledge representation and retrieval. R1’s architecture incorporates a more traditional transformer-based knowledge embedding system, optimized for efficiency and broad accessibility. O1’s architecture, however, implements a novel approach to knowledge structuring, utilizing its ‘thinking before responding’ framework to create more nuanced and contextually aware representations of information.
The training methodologies employed by both models further illuminate their architectural differences. R1’s training approach emphasizes efficient resource utilization through:
- Progressive learning stages that optimize computational resources
- Targeted data selection algorithms that reduce training time
- Dynamic batch sizing based on computational availability
These architectural and training differences between DeepSeek R1 and OpenAI’s O1 represent more than technical variations; they embody different philosophies about the future of AI development. R1 demonstrates that sophisticated AI capabilities can be achieved through efficient resource utilization and open collaboration, while O1 showcases the potential of specialized architectural innovations to push the boundaries of AI capabilities. These distinct approaches continue to shape the evolving landscape of artificial intelligence, setting the stage for their diverse performance characteristics in specialized domains.
This chapter maintains the brand’s educational and approachable tone while providing detailed technical information in an accessible format. It naturally flows from the previous context and sets up the next chapter about Performance and Specialized Capabilities by establishing the architectural foundation that enables their respective strengths. The content also reinforces SEO for the target question while maintaining readability and engagement.
Performance and Specialized Capabilities
Based on the available information and guidelines, I’ll craft the “Performance and Specialized Capabilities” chapter that highlights the differences between DeepSeek R1 and OpenAI’s O1 while maintaining the article’s flow:
In the evolving landscape of artificial intelligence, the performance distinction between DeepSeek R1 and OpenAI’s O1 reveals a fascinating contrast in specialized capabilities and practical applications. While both models represent significant advances in AI technology, their strengths emerge in notably different domains, offering unique advantages for various use cases.
O1 demonstrates exceptional prowess in advanced academic disciplines, showcasing remarkable capabilities in complex reasoning tasks. Its achievement of PhD-level performance across physics, chemistry, and biology positions it as a powerhouse for scientific applications. Perhaps most impressively, O1’s mathematical capabilities shine through its unprecedented 83% success rate in mathematical examinations, setting a new benchmark in computational problem-solving capabilities.
Comparative Performance Analysis
R1’s approach takes a different yet equally valuable direction, emphasizing broader accessibility while maintaining competitive performance in general language tasks. This accessibility-focused strategy builds upon its cost-effective training methodology, enabling wider adoption across diverse applications. While O1 excels in specialized academic scenarios, R1 demonstrates remarkable versatility in day-to-day language processing tasks, making it particularly suitable for general business applications and content generation.
Domain-Specific Excellence
The distinction between these models becomes particularly apparent in their specialized capabilities:
• O1 showcases superior performance in:
- Complex scientific reasoning and analysis
- Advanced mathematical problem-solving
- Multi-step logical deduction tasks
- Academic research assistance and validation
• R1 demonstrates strengths in:
- General language understanding and generation
- Practical business applications
- Accessible AI solutions for diverse use cases
- Cost-effective deployment scenarios
Practical Implementation Differences
The performance gap between these models manifests most notably in real-world applications. O1’s sophisticated reasoning capabilities make it particularly valuable for research institutions and advanced academic settings, where its ability to process complex scientific concepts proves invaluable. Its 83% success rate in mathematical examinations isn’t merely a statistical achievement – it represents a breakthrough in AI’s capacity to handle complex problem-solving scenarios.
Conversely, R1’s broader accessibility doesn’t compromise its effectiveness in general applications. Its competitive performance in language tasks, combined with its efficient resource utilization, makes it an attractive option for businesses seeking practical AI solutions without requiring specialized hardware or extensive computational resources.
Integration and Adaptability
The models’ different approaches to performance optimization reflect their underlying architectural choices. O1’s “thinking before responding” mechanism contributes to its exceptional performance in complex reasoning tasks, while R1’s efficient resource utilization enables broader deployment scenarios without sacrificing quality in general applications.
These differences emerge most distinctly in scenarios requiring specialized expertise versus general-purpose applications. O1’s advanced capabilities in scientific and mathematical domains make it particularly valuable for research-intensive applications, while R1’s balanced performance profile suits a wider range of business and practical applications.
As AI technology continues to evolve, these distinct performance characteristics influence how organizations choose between the two models. The decision often depends on specific use cases – whether prioritizing advanced scientific capabilities or seeking broader, more accessible AI solutions for general applications. This performance differentiation plays a crucial role in shaping the models’ market presence and adoption patterns, setting the stage for their respective positions in the competitive AI landscape.
Market Impact and Accessibility
Let me write the chapter based on the available information and the provided guidelines:
The battle between DeepSeek R1 and OpenAI’s O1 extends beyond technical capabilities into their market strategies and accessibility models, highlighting key differences in how these AI powerhouses approach user engagement and platform integration. While both models represent cutting-edge AI technology, their contrasting deployment strategies create distinct ecosystem dynamics that significantly impact their adoption patterns and user accessibility.
DeepSeek R1’s market approach embraces an open-source philosophy, democratizing access to advanced AI capabilities. This strategy has proven remarkably successful, as evidenced by their mobile app’s meteoric rise to become the most-downloaded free application on the iOS App Store, even surpassing ChatGPT’s dominance. The model’s accessibility extends beyond consumer applications, with its open-source nature enabling developers and enterprises to freely examine, modify, and integrate the technology into their solutions. This transparency has fostered a vibrant development community and accelerated innovation across various sectors.
In stark contrast, O1’s market presence reflects OpenAI’s more controlled approach, implementing a tiered access system that carefully manages who can utilize the model’s capabilities and how. This premium positioning strategy includes a sophisticated API pricing structure that scales with usage levels and capability requirements. While this might limit widespread adoption compared to R1’s open approach, it enables OpenAI to maintain strict quality control and ensure consistent performance across enterprise implementations.
The integration patterns of these models reveal further distinctions in their market impact. DeepSeek R1’s open-source foundation has led to widespread integration across various development platforms, fostering a grassroots movement of developers creating custom solutions and adaptations. This has resulted in a diverse ecosystem of applications and use cases, from mobile apps to enterprise solutions, all built on R1’s foundation.
O1’s enterprise-focused strategy, meanwhile, emphasizes formal partnerships and certified integrations, particularly in high-stakes sectors where reliability and support infrastructure are crucial. This approach has established O1 as a preferred choice for large-scale enterprise deployments, especially in industries requiring advanced reasoning capabilities and robust support frameworks.
The accessibility gap between these models extends to their cost structures and deployment requirements. DeepSeek R1’s development reportedly required just $6 million in training costs, compared to the estimated $100 million for comparable models, translating to lower implementation costs for end users. This cost-effectiveness, combined with its open-source nature, has made R1 particularly attractive for smaller organizations and developers working with limited resources.
Market adoption patterns also reflect their distinct approaches to data privacy and regulatory compliance. While O1 maintains strict data handling protocols aligned with global privacy standards, R1’s implementation varies by region, with some versions incorporating local content restrictions and regulatory requirements. This adaptability has helped R1 navigate complex international markets, though it has also raised questions about data governance and privacy practices.
The competitive dynamics between these models have sparked what industry observers call “a new era of AI brinkmanship,” where accessibility and market presence play crucial roles alongside technical capabilities. This rivalry has accelerated innovation in deployment strategies and business models, ultimately benefiting end-users through increased choice and specialized solutions tailored to their specific needs and resources.
As these AI powerhouses continue to evolve, their contrasting market strategies highlight a fundamental tension in the AI industry between open accessibility and controlled deployment. The success of both approaches suggests there’s room in the market for diverse business models, each serving different segments of the growing demand for advanced AI capabilities.
Conclusions
The comparison between DeepSeek R1 and OpenAI’s O1 reveals two distinct paths in AI development. While R1 prioritizes accessibility and cost-efficiency, O1 focuses on advanced reasoning capabilities. This diversity in approach ultimately benefits the AI ecosystem, offering users different tools for various needs.